How Invert uses batch context to make your data valuable—instantly
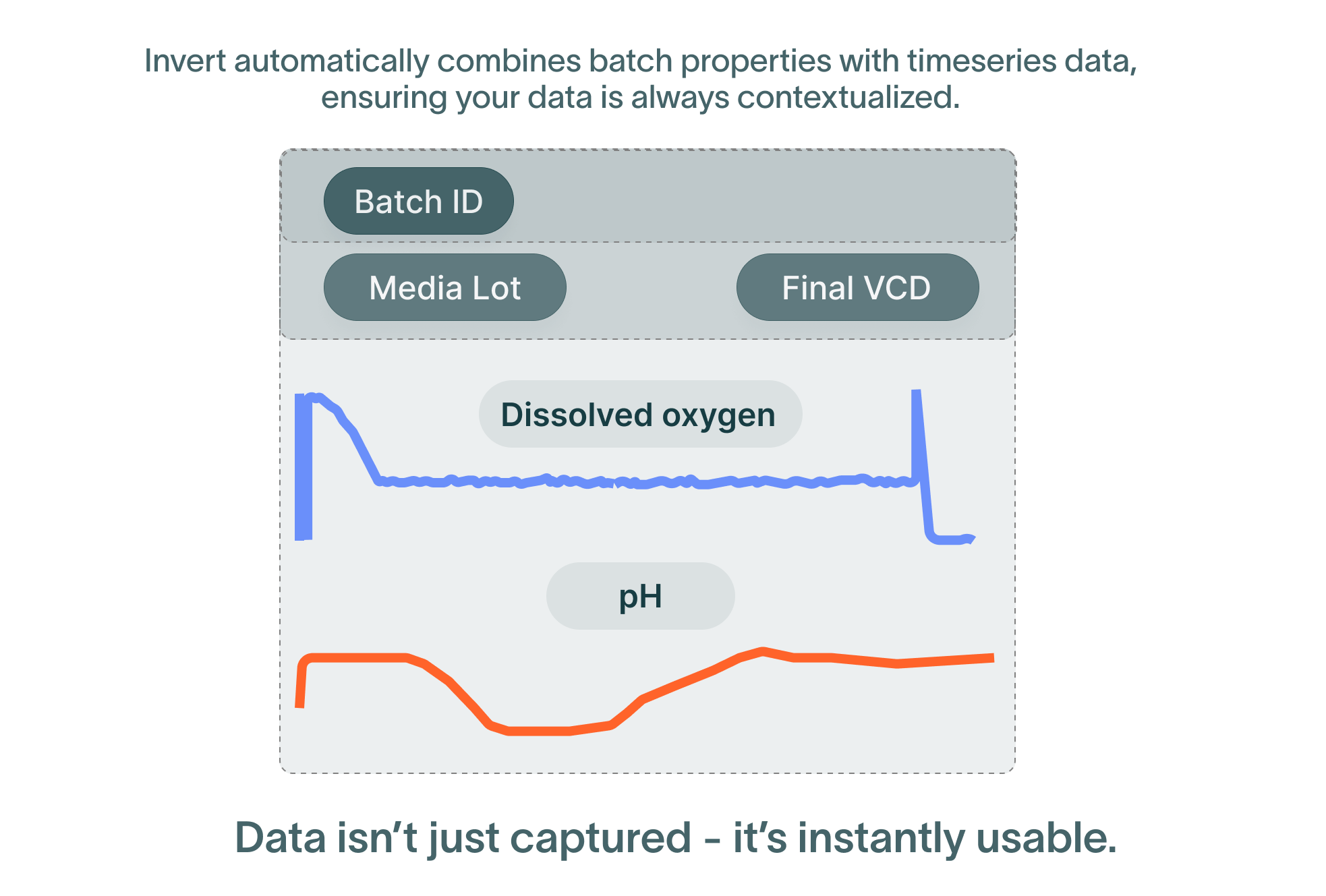
Bioprocess data can be challenging to capture, and perhaps even harder to interpret. Data collection must be set up, whether it’s file uploads from a BioFlo® 320 or streaming data from an Ambr® 250 OPC UA interface. Streams of data from multiple systems must be mapped to scientific domains like pH and temperature.
At Invert, we work with many types and configurations of process equipment—and we’re familiar with the challenge of understanding all the data that they generate. With thousands of data points arriving every minute, routine troubleshooting and monitoring of processes is complex. The potential answers to process challenges like cell growth delays or product aggregation are concealed within featureless traces of scrolling timeseries data. Bioprocess engineers have to export data and surgically reformat it in Excel, column by column, into something useful—every single time they’re trying to resolve production issues.
Batch manufacturing adds complexity
Biopharmaceutical manufacturing is commonly done in batches, unlike other chemical industries that operate continuous processes1. Batch manufacturing appears to simplify things by breaking up processing into discrete chunks. In reality, it adds complexity—timeseries data only makes sense in relation to other data.
The data generated in batch manufacturing exists in two main forms. The first form is batch-level data that describes an output or input for the entire activity, such as the inoculation time, final titers or media lot numbers. The second form is timeseries data, measuring process parameters such as temperature or pH. Troubleshooting and optimizing processes requires connecting these two forms of data. For example, did fluctuations in temperature affect the final titer of a batch? It depends on batch context: where and when in the batch were these fluctuations occurring?
Without batch context, timeseries data can show how certain parameters changed over time, but it cannot tell us how these changes will impact the process. Batch context allows us to derive meaning from data: a higher pH might be acceptable at inoculation, but unacceptable at transfection. This exists at multiple levels—first, is the data part of a batch at all? Some systems collect data even when not in use. If the data is part of a batch, it needs operation and phase context—where exactly did it occur? Lastly, the data needs to be normalized against a start time, so it can be analyzed alongside a golden batch or other reference data.
Many systems are not “batch aware”
Bioprocess development involves an array of data-generating equipment, from bioreactors, to purification skids, to scales and benchtop pH probes. All of these systems measure and report physical parameters. However, they usually only know what material they’re measuring if an embedded software interface captures that data from the user. While some systems have this, not all do. And the ones that do are subject to human error—batch IDs may be entered incorrectly, at the wrong time, or forgotten entirely.
Even if this all works perfectly, users must still write queries to pull out data tagged with the right batch ID and normalize it from absolute to relative time. Many process engineers find that this workflow is brittle enough to avoid this functionality even if it’s available, and opt to organize data post hoc. Custom automation might help, but requires custom configuration and takes time to update.
Bioprocess teams can spend dozens of hours every week exporting data and manually cutting it up to assign it to the batches they care about—taking part of a timeseries and copying it into one spreadsheet, and another part and copying it into another spreadsheet. Often this happens in the slivers of time between other, more urgent activities, resulting in data only being reviewed once or twice a week.
Invert applies context on the fly
Invert is built on the fact that batch-contextualized data is the essential input for bioprocess analysis. We’ve built a way for users to easily add batch context, whether it’s labeling data after a run or pre-programming an integration to inject that context on the fly.
For users, this means no new tags need to be configured. You don’t need to designate triggers to catch batch starts and ends either (though we do that too, for parts of our integration portfolio). Instead, simply select the relevant runs and data and assign the relevant time window—regardless if that window was in the past, the future, or happening right now.
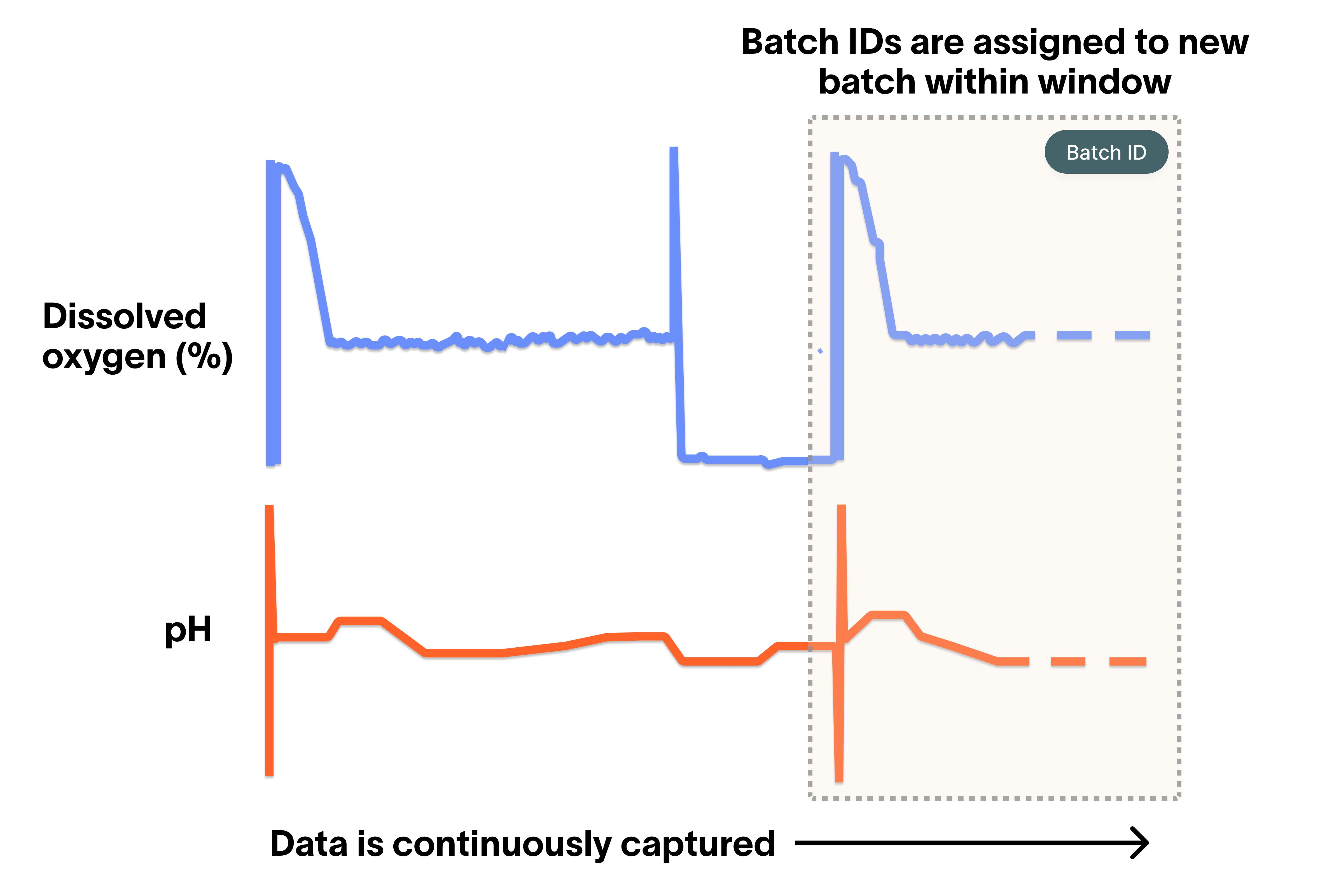
If that batch is in the past, Invert assigns the relevant data to the batch immediately.
If the batch is in the future, Invert creates a trigger which will route incoming data into the desired batch when it starts, and continue until the batch completes.
You can even assign data to a batch while it’s in-flight—Invert both assigns the already-ingested data and directs incoming data to the batch.
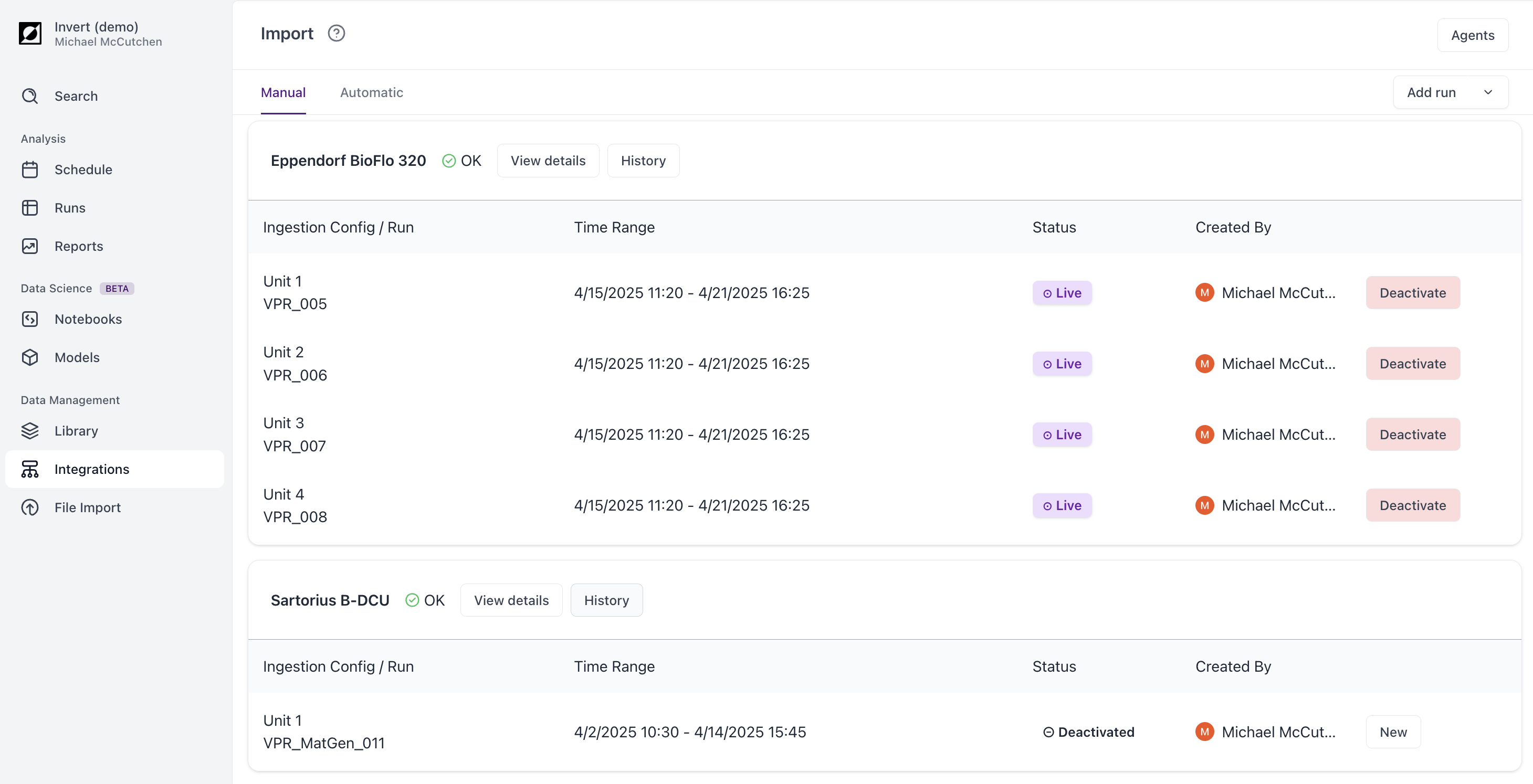
With large volumes of data, mistakes are inevitable. If the wrong batch ID is entered or the wrong time window selected, the consequences are hours in Excel of untangling fragmented, mislabeled data. With Invert, it’s a simple fix. Just archive the old data, and re-assign the data with a click.
Once assigned, Invert always stores data within its batch context. If you export your data or access it through the Invert API, this association is preserved.
For data already in a historian, this works similarly—Invert layers batch context on top, just like when it's streaming directly from equipment.
Batch context makes data valuable
Invert focuses not just on capturing data, but making it instantly useful. Batch context is part of that puzzle—it converts raw sensor readings into something you can interpret, right now. Old data benefits as well. When you return to it, it’s contextualized and ready to use.
Currently, only a fraction of biomanufacturing data is truly used though it is expensive to generate, because contextualization is challenging to do at scale. Terabytes of data remain trapped in spreadsheets and databases. By automatically positioning all biomanufacturing data in its appropriate context, we unlock the knowledge contained within.
- Khanal, Ohnmar, and Abraham M. Lenhoff. “Developments and Opportunities in Continuous Biopharmaceutical Manufacturing.” mAbs, vol. 13, no. (1 Jan, 2021), p: e19036641- e1903664-13. https://doi.org/10.1080/19420862.2021.1903664
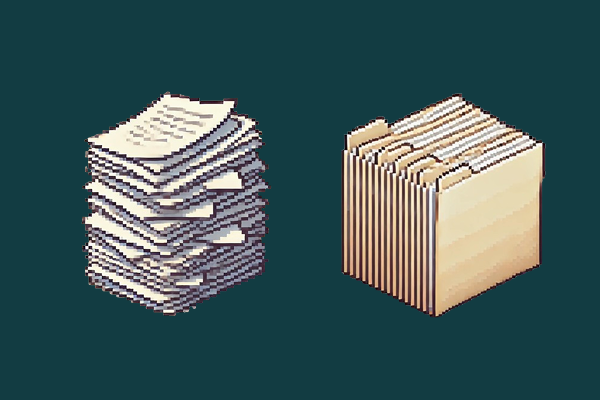
Why ELNs and LIMS are not enough for PD teams
One of the questions we’re asked frequently is whether Invert is an ELN or LIMS—our answer is that Invert captures both systems’ strengths and fixes their weaknesses. We’ll discuss the trade-offs and shortcomings of ELN and LIMS when it comes to bioprocess data to explain how.
Read More ↗.png)
How Invert uses meta-learning to leverage old bioprocess data
With meta-learning techniques, Invert’s ML team was able to reduce new data needs by as much as 90% by leveraging old data from different, but loosely related, bioprocess programs.
Read More ↗.png)
Pioneers in Bioprocessing: Q&A with Biopharma Executive, Steven Lang, Ph.D.
Biotech and synthetic biology companies have much to do. Handling everything in-house—from strain design to process development—can stretch funding and resources thin.
Read More ↗